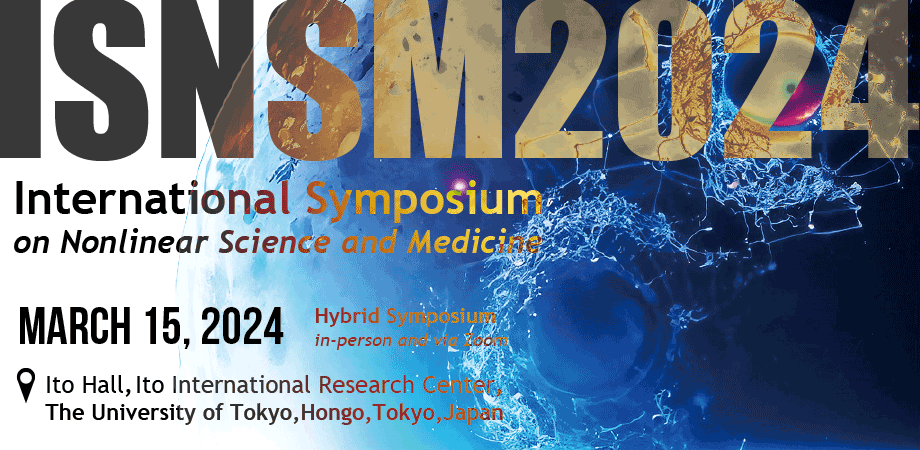
Poster Presentations Abstracts
No. | 1 |
---|---|
Poster Title | Causal Control of Phenotype and State Transition |
Authors | Chengming Zhang, Zexin Chen, Deyu Cai, Kazuyuki Aihara, and Luonan Chen |
Abstract | Understanding and purposefully manipulating cell states or phenotype transitions remains a persistent challenge in the field of biology. In this study, we introduce CauFinder, an innovative interpretive framework that employs neural networks to emulate the hierarchical control structure inherent in biological systems. The primary objective is to identify a subset of features that exert causal influences during state or phenotype transitions. By executing the "do" operation and optimizing causal information flow metrics, we effectively isolate causal factors within the latent space, enabling the generative model to provide concise, precise, and causally informed explanations. Furthermore, after identifying causal features using SHAP feature selection, we employ optimization techniques to manipulate these features, thereby facilitating state transitions. Through various simulated and real-world application scenarios, we have demonstrated the effectiveness and applicability of our approach. |
No. | 2 |
---|---|
Poster Title | Early Warning Signal for Random Dynamical Systems with Bounded Noise |
Authors | Wei Hao Tey, Guillermo Olicón Méndez, Kazuyuki Aihara |
Abstract | Critical transitions in a dynamical system have been a topic of discussion in different disciplines. The understanding of its mechanisms and potential early warning signals are important for predictions and plannings of the sudden change. Traditional early warning signal is based on the concept of critical slowing down where variance of the data increases as the system approaches critical transition. However, if the noise magnitude is large, there exists some examples where monitoring variance is not sufficient for detecting critical transition in data. We introduce an additive bounded noise model, where the random terms in a random dynamical system are additive and bounded by an epsilon ball. In this framework, we illustrate that a potential early warning signal is by approximating derivative of the extremal map using the data near to the boundary of the sample data. This is achieved by utilizing the asymptotic equation of the stationary distribution of the random dynamical system and fitting them with the tail of histogram of the sample data. |
No. | 3 |
---|---|
Poster Title | Causal inference Based on Perturbed Embedding Entropy |
Authors | Yang Li, Jifan Shi, Kazuyuki Aihara |
Abstract | Inference of causal relationships has a great potential in the analysis of various network systems. Compared with traditional methods such as Granger causality and transfer entropy, the methods based on time-delayed embedding of dynamical systems have been attracting increasing attention. Here we propose an indicator for the cauality from variable x to y, perturbed embedding entropy, which calculates the entropy loss in ΔX given ΔY, where ΔX and ΔY denote deviation in delayed coordinate X of x and that of y, respectively. Numerical investigation on simulated data (from chaotic neural networks) and real-world data (Japan Covid19 dataset) demonstrate the effectiveness and usefulness of this method. |
No. | 4 |
---|---|
Poster Title | Effect of Time Series Length on Estimation of Mouse Pupillometry Dynamics' Characteristics |
Authors | Nina Sviridova, Pietro Artoni, Michela Fagiolini, Takao K. Hensch, and Kazuyuki Aihara |
Abstract | Mouse models' spontaneous pupil size fluctuations are noninvasive data used in applied studies aiming at the early detection of neurodevelopmental spectrum disorders. While pupillometry data are highly valuable and used in various studies, its complex dynamics properties are still to be thoroughly investigated. Various studies use nonlinear time series analysis methods to investigate the dynamical characteristics of complex biomedical signals, such as pupillometry. While this analysis toolkit proved useful in unveiling dynamical features, it imposes various requirements on the experimental signals, such as signal length, sampling rate, etc. Collecting sufficiently long experimental data from animals might be challenging, and as a result, in many cases, signals recorded in the experiment might be of limited length. This study aims to investigate the effect of pupillometry signal length on the estimation of its dynamical characteristics and to find the minimal length of the time series that ensures the reliability of its results. |
No. | 5 |
---|---|
Poster Title | Identification of atrial fibrillation in ECG using machine learning methods |
Authors | K. Sakai, T. Saji, K. Takeda, H. Kitajima, M. Ishizawa, T. Minamino |
Abstract | Atrial fibrillation is the most commonly encountered clinical arrhythmia, which is caused by a disturbance of the electrical signals flowing in the atria. Atrial fibrillation is not immediately dangerous, but it is associated with an increased risk of myocardial infarction, heart failure, stroke, dementia, chronic kidney disease. Therefore, early detection of atrial fibrillation is important. In this study, machine learning methods(Convolutional neural networks and Vision Transformer) were used to detect atrial fibrillation in the electrocardiogram (ECG). We tried three types of input image data: the ECG waveforms, wavelet-transformed images, recurrence plots of the waveforms. Stratified k-fold cross-validation was used for training and validation, and the average of five times was taken. The best result was the case of recurrence plots: accuracy of 97.12%, recall of 97.52%, and specificity of 96.70%. Now, we are trying to mini-batch optimization for unbalanced data and building more accurate models using ensemble learning. In the future, we plan to deal with the multiple classification problem, including arrhythmias other than atrial fibrillation. |
No. | 6 |
---|---|
Poster Title | LSTM-based anomaly detection of pulse waves for identifying atrial fibrillation |
Authors | Kentaro Takeda, Hiroyuki Kitajima, Makoto Ishizawa, and Tetsuo Mimamino |
Abstract | A detection method for atrial fibrillation (AF) in pulse waves obtained with a blood pressure monitor is presented. In the presented method, long short-term memory (LSTM) is used for time series forecasting of sinus rhythm (SR), and its forecast anomalies are detected as AF. The presented method achieved high accuracy with both high specificity and sensitivity. |
No. | 7 |
---|---|
Poster Title | Approximating nonlinear dynamics on networks by sentinel nodes |
Authors | Neil G. MacLaren, Baruch Barzel, Naoki Masuda |
Abstract | Many systems, including disease progression and transmission, can undergo sudden, drastic changes. These changes often involve many variables and can be difficult to predict. Several dimensionality reduction methods for nonlinear dynamics on networks assume that nodes with the most connections are the most important in approximating the state of the system. While these nodes may be causally important in a dynamical process, they may also be outliers in terms of current system state, biasing the approximation. Given a model of the system which we have solved numerically over a critical range of a control parameter, we select a small number of sentinel nodes by combinatorial simulated annealing. The mean state of the sentinel nodes should approximate the unweighted mean state of all nodes. We find that our method achieves reasonable accuracy across several networks and dynamical models and is robust to some uncertainty in model parameters. Over many independent runs, our algorithm avoids hub nodes---precisely the nodes assigned the greatest importance by previous methods---and instead includes a mix of small and large degree nodes in the vicinity of the mean degree. Node sets with the lowest approximation error tend to include nodes whose neighbors also have moderate degree. If we assign weights to the sentinel nodes using quadratic programming optimization during simulated annealing, we find that our algorithm behaves similarly in terms of node selection as when we do not assign weights, and additionally tends to assign maximum weight to nodes near the mean degree. We thus conclude that although hub nodes are important in the temporal evolution of dynamics on networks, the dynamical state of a network may be best approximated by average nodes. |
No. | 8 |
---|---|
Poster Title | Dynamical Network Biomarker Theory for Hierarchical Networks |
Authors | Hampei Sasahara, Yuto Saito, Jun-ichi Imura |
Abstract | Dynamical Network Biomarkers (DNB) theory has been proposed as a method for detecting diseases at a very early stage. The progression of a disease can be regarded as a bifurcation phenomenon of the underlying dynamical system associated with the corresponding gene network. By identifying large fluctuations of the gene expression level occurring just before the bifurcation, we can detect the pre-disease stage without identifying the mathematical model of the dynamical system. However, the existing DNB theory mainly focuses on a single gene network representing averaged dynamics of multiple cells. In this study, we consider extending DNB theory to a cell-to-cell network composed of health and disease cells. It is numerically shown that the pre-disease stage appears when the number of disease cells increases. |
No. | 9 |
---|---|
Poster Title | Theory of restabilisation of high-dimensional data-driven network systems in very early treatment |
Authors | Xun Shen, Hampei Sasahara, Jun-ichi Imura, Makito Oku, Kazuyuki Aihara |
Abstract | The Dynamical Network Biomarkers (DNBs) theory has been proposed to detect early-warning signals of critical transitions in gene regulatory networks only with High-Dimension Low-Sample-Size (HDLSS) data of the system state. Towards giving a theoretical foundation for early medical treatment, this paper proposes a data-driven approach for the re-stabilization of gene regulatory networks based on HDLSS data. In the proposed re-stabilization method, only the diagonal elements of the system matrix need to be adjusted. Namely, only the self-feedback loops of mRNA expression for genes are intervened in, which reduces the complexity of the early medical treatment based on gene regulation and makes it practical to be implemented. The proposed re-stabilization method is generalized to the systems with either saddle-node bifurcation or Hopf bifurcation. Numerical simulations have been implemented to validate the effectiveness of the proposed method. |
No. | 10 |
---|---|
Poster Title | A modified EKF approach to modeling GRNs via short gene expression time series |
Authors | Zhenhui Xu and Jun-ichi Imura |
Abstract | In this study, a modified extended Kalman filter (EKF) method is developed to model the gene regulatory network from small size gene data. After specifying the model structure, the modified EKF method is applied to identify parameters of gene expression levels. The proposed algorithm consists of a double-loop iteration. The inner loop is the same as the traditional EFK iteration, while the outer loop allows the measurement data to be reused, which greatly reduces the number of observation data requirement. It is shown that the modified EKF algorithm can identify a large number of parameters by using a small amount of observations. A gene expression data set is employed to demonstrate the effectiveness of the algorithm. |
No. | 11 |
---|---|
Poster Title | Data Analysis to Investigate the Process of EMT in NMuMG Mouse Mammary Epithelial cells |
Authors | A.S.M. Bakibillah, H. Sasahara, X. Zhenhui, J. Imura |
Abstract | Epithelial-Mesenchymal Transition (EMT) is a process by which epithelial cells lose their cell polarity and cell–cell adhesion, and gain migratory and invasive properties to become mesenchymal stem cells. EMT occurs in embryonic development, wound healing, organ fibrosis, and initiation of metastasis in cancer progression. A strong promoter of EMT is TGF-β. In this work, we analyze TGF-β induced EMT data measured by DNA microarray over time after stimulation with TGF-β at 7 time points. We perform principal component analysis (PCA), differential expression gene (DEG) analysis, and dynamic network biomarker (DNB) analysis on the data. From the analysis, we obtain some important genes that could be the possible biomarkers of EMT in the pre-disease stage. |
No. | 12 |
---|---|
Poster Title | Decrease in natural frequency as an overlooked early warning signal |
Author | Makito Oku |
Abstract | Several early warning signals such as an increase in fluctuations have been proposed to predict imminent critical transitions in various complex systems in ecology, climatology, life sciences, and so on. Many early warning signals are also theoretically proven to emerge near a saddle-node or Hopf bifurcation under certain conditions. However, early warning signals that emerge once but disappear again very close to the bifurcation point seem to be overlooked. One example is a decrease in natural frequency. This phenomenon occurs when a pair of complex conjugate eigenvalues get closer to each other. After they collide, they become a pair of real eigenvalues. After that, one of them moves toward the origin, resulting in a saddle-node bifurcation. Results of numerical simulations indicate that this phenomenon easily occurs in a linear dynamical system when a non-diagonal element of the system matrix changes continuously. This phenomenon may extend the concept of critical slowing down and could be used for detecting pre-disease states when vital data from high-frequency measurements are available. |
No. | 13 |
---|---|
Poster Title | Discrimination of Multiple Myeloma and Precancerous State MGUS by Raman Microscopy |
Authors | Akinori Taketani, Shota Koshiyama, Jun Tahara, Keiichi Koizumi, Yusuke Oshima, Takayuki Haruki, Shota Yonezawa, Kohei Kunimoto, Daiki Kirigaya, Akinori Wada, Tsutomu Sato, Shigeru Saito |
Abstract | Multiple myeloma (MM) is a malignant disease in which plasma cells become "cancerous" and grow abnormally in the bone marrow, producing large amounts of useless antibody M protein, which causes abnormalities throughout the body. MM has a precancerous state Monoclonal Gammopathy of Undetermined Significance (MGUS) before becoming tumors. The MGUS state is characterized by abnormal proteins in the serum, but in small amounts and without organ damage, and the patient is followed without treatment. In the current situation, MM is differentiated based on bone marrow cell staining to determine whether they are in an MGUS or MM when the ratio of normal cells reaches 10%, and the need for anticancer drug treatment is determined. Therefore, there is a need for the development of a more accurate differentiation method. We were developing a new discrimination method for MM and MGUS using Raman microscopy. Raman spectroscopy is a non-destructive method for obtaining molecular information from cells. This study aimed to identify and clarify differences in MM and MGUS plasma cells' structures and conditions by measuring non-destructively with Raman microscopy. In this study, we measured Raman spectra of 35 MM, 25 MGUS and 38 normal patient samples. The results of partial least squares (PLS), a form of multivariate analysis, suggested that MM, MGUS, and normal samples differ in lipid and DNA composition. |
No. | 14 |
---|---|
Poster Title | Identification of the Mebyo genes for metabolic syndrome using the model organisms |
Authors | Kazutaka Akagi, Yingjie Jin, Makito Oku, Jun-ichi Imura, Keiichi Koizumi, Shigeru Saito |
Abstract | Contemporary healthcare predominantly focuses on therapeutic interventions initiated post-disease onset, often requiring prolonged treatment periods and substantial medical expenses. Consequently, there is a pressing need to accurately identify and intervene in the pre-disease stage, known as "Mebyo", to achieve ultra-early prevention of illnesses. However, the scientific definition of "Mebyo" remains elusive, necessitating the development of methodologies and experimental verification to precisely describe this concept. In our study, we aimed to elucidate the pre-disease state in the onset of metabolic syndrome using a spontaneous model of metabolic syndrome in mice (TSOD mice). We performed microarray analysis using the adipose tissue of TSOD mice at the stage of pre-disease onset and applied Dynamical Network Biomarkers (DNB) theory to analyze the data. Successfully detecting fluctuations in gene expression with high correlation index, we defined this point as the "Mebyo" stage in our mouse model. Furthermore, utilizing DNB theory, we narrowed down the Mebyo genes (DNB genes) to a set of 18 genes predicted to have a high efficacy in genetic interventions using control theory. Intriguingly, these genes did not include those previously reported in association with metabolic disorders. To elucidate the metabolic roles of these genes, we took advantage of the genetically manipulable fruit fly, Drosophila melanogaster, and conducted RNAi screening. Knocking down the Drosophila orthologs of the DNB genes specifically in the adipose tissue, we assessed the metabolic impact through starvation resistance tests. We found two genes, which shows large impact on metabolic functions. This presentation discusses these findings, future prospective, and the utility of DNB theory in combination with Drosophila research model. |
No. | 15 |
---|---|
Poster Title | Development of a Quantitative Prognostic Approach for Acute Liver Failure |
Author | Raiki Yoshimura |
Abstract | As observed in recent cases of severe COVID-19, detecting biomarkers that indicate early signs in acute diseases with dramatic changes in condition is an urgent challenge for predicting future pathological changes. In this study, using clinical data from a disease called acute liver failure, where the survival rate significantly decreases after onset, we developed an approach to predict changes in condition and prognosis. Specifically, by analyzing patient blood test data using supervised machine learning, we identified factors that could serve as biomarkers for predicting changes in condition. Developing a mathematical model describing the time series changes of identified factors allowed us to extract parameters explaining the time changes for each patient. Furthermore, by constructing a machine learning model to predict patient parameters from admission data, we successfully developed an early prognosis prediction model. The approach developed in this study is expected to be utilized in clinical medicine as a tool for early response decision-making. |
No. | 16 |
---|---|
Poster Title | Quantitative evaluation of mucosal antibodies' role in inhibiting infectious SARS-CoV-2 shedding |
Authors | Takara Nishiyama, Sho Miyamoto, Park Hyeongki, Shingo Iwami |
Abstract | As the COVID-19 pandemic is coming to an end, there is a crucial need to develop technologies for managing future pandemics, particularly those caused by emerging Variants of Concern (VOCs) and other respiratory viruses. Despite the effectiveness of mRNA vaccines in blocking SARS-CoV-2 entry, their role in preventing viral shedding from infected individuals is less clear. Mucosal immunity, essential in respiratory infections, might be key in reducing viral shedding and human transmission. However, understanding of mucosal immunity's role in this context is limited. This study aims to quantify the impact of mucosal antibodies on viral shedding suppression using a mathematical model reconstructing SARS-CoV-2 dynamics. Nasopharyngeal swab data from individuals infected with SARS-CoV-2 omicron variants were analyzed. These swabs were collected in a cohort study by the Japanese Ministry of Health, Labor and Welfare. Utilizing mathematical models, we extracted quantitative individual-level data on mucosal antibody and viral dynamics. We found a significant correlation between virus shedding dynamics and mucosal antibody responses post-infection. Notably, secretory IgA (S-IgA) was instrumental in suppressing infectious virus shedding, highlighting the importance of its rapid production for control. These findings suggest a potential strategy to control the spread of respiratory viral infections, emphasizing the role of mucosal immunity, especially S-IgA, in managing viral shedding and transmission. |
No. | 17 |
---|---|
Poster Title | Detection of the changes in the depression state under the COVID-19 pandemic by using the energy landscape analysis (ELA) |
Authors | Daiki Tatematsu, Naotoshi Nakamura, Shinsuke Koike, Shingo Iwami |
Abstract | The COVID-19 pandemic changed our lifestyles. It is expected that the changes in depression state also occurred because of these changes. In this study, we used the questionnaire that asked high school students in Tokyo about their depression states before, during, and after the period of the COVID-19 pandemic and analyzed the group characteristics of changes in depression state as a landscape using energy landscape analysis (ELA), a method of multidimensional (time-series) data analysis. As a result, we were able to quantitatively confirm the depression state changes as the energy barriers. We were also able to detect how the energy barrier changed in the COVID-19 pandemic and found that the energy barrier was relatively high in the COVID-19 pandemic and that it was difficult to change to a depression state. These results are consistent with those of previous studies and suggest that ELA can be used for the psychiatric questionnaires analysis. |
No. | 18 |
---|---|
Poster Title | Identifying distinct neuroanatomical subtypes of Major depression disorder and Schizophrenia |
Authors | Yinghan Zhu, Norihide Maikusa, Kiyoto Kasai, Shinsuke Koike |
Abstract | Prior neuroimaging studies primarily focused on investigating neuroanatomical abnormalities in mental disorders such as major depression disorder (MDD) or schizophrenia (SCZ) use binary case-control approach.Brain structural abnormalities such as cortical thinning and subcortical volume increasing were reported in MDD or SCZ compare to heathy controls (HCs). We investigated neuroanatomical subtypes in multi-disorder, multi-site multi-protocol , using semi-supervised machine learning methods heterogeneity through discriminative analysis (HYDRA) to discover variations of anatomical alterations within disorders. T1-weighted structural brain MRI scans from patients with MDD (n=544), SCZ (n= 176), and 1,819 HCs, were obtained from 9 sites. Regional cortical thickness (CT), surface area (SA) and subcortical volumes (SV) served as features in building the classifier differentiating disorder subtypes from HCs. We used traveling subjects dataset to harmonize measures of CT, SA and SV data. Next, we fitted general addictive models to only the HC data (n=1148) to estimate non-linear effects of age and sex for every structural feature; then we applied the fitted GAMs to obtain non-linear age- and sex-corrected features. Individuals those who were younger than 65 years old (MDD, n=445; SCZ, n=158) and HC (n=599) data served as training, test, and external validation datasets. Two distinct neuroanatomical subtypes were found for MDD and SCZ. In differentiating Subtype 1 or subtype 2 from HCs, the accuracy of each classifier was higher than those built for disorder without subtypes (MDD subtype1 vs HCs 79%; MDD subtype2 vs HCs 76%; MDD vs HCs 67%; SCZ subtype1 vs HCs 83%; SCZ subtype2 vs HCs 86%; SCZ vs HCs 75%). Indicating that by identifying subtypes of MDD and SCZ improved the performance in differentiating those from HCs. Discovering of neuroanatomical subtypes of MDD and SCZ may be helpful to identify their prognosis and underlying neuropathological processes. |
No. | 19 |
---|---|
Poster Title | Multi-site large sample MRI dataset for adolescent development and psychiatric disorders |
Author | Shinsuke Koike |
Abstract | It is well-known about the heterogeneity of psychiatric disorders and each spectrum can include two or more pathophysiological backgrounds, a part of which in turn can be shared with some of other spectra. Recent large-scale MRI studies have found the MRI-based subtypes in schizophrenia (Okada et al. Mol Psychiatry 2023; Chand et al. Brain 2020). Therefore, when seeing brain characteristics into the subtyping level, we can look at a new insight for multiple psychiatric disorders and adolescent development. When using multi-site dataset, we need to pay attention to a measurement bias from MRI machines and protocols, since the measurement bias is greater effect size compared to disease-related difference from healthy controls (Maikusa et al. Hum Brain Mapp 2021; Koike et al. NeuroImage Clin 2021; Kurokawa et al. NeuroImage 2021; Yamashita et al. PLOS Biol 2020). Recent Japanese MRI projects have targeted for cross-disorder dataset for neuropsychiatric disorders and high-quality harmonization techniques using a traveling subject method (Koike et al. NeuroImage Clin 2021). As of October 2023, we harmonized more than 3,000 samples of people with schizophrenia, major depressive disorders, bipolar disorders, and autism spectrum disorders as well as clinical high risk for psychosis and general adolescents. Here, I intend to introduce about the ongoing collaborative studies for neuropsychiatric disorders and harmonization techniques. |
No. | 20 |
---|---|
Poster Title | On the uncertainty principle and geometrization for spike coding models |
Authors | Xun Li, Lang Cao, Kazuyuki Aihara |
Abstract | Spiking computation is a prominent branch of artificial intelligence that embraces the advantages of low energy consumption due to its massively parallel, event-driven nature. Theoretical understanding of how information can be coded and represented by sequences of spikes is a first step for the design of spike-based computing systems towards their fully exploited potential. In studying models of spike coding from a physicist's perspective, the familiar uncertainty principle can be drawn on the energy-time domain, giving a lower bound on the product of the variances of spike counts and spike timing in the codes required by preservation of information. Thus, we term a minimum uncertainty scheme the coherent coding. It can be obtained from the action principle by minimizing the Lagrangian, the difference between the kinetic and potential energies of the coding, which correspond, respectively, to the complexity of the spike codes from their temporal and rate components. Motivated by this, we further postulate a general geometrization framework for spike coding models, where information is assumed to be represented by curved paths in a high-dimensional manifold, and spike codes are obtained by discretizing the paths with equally spaced points and then projecting into a one-dimensional time axis. Finally, we illustrate our model with the application of word embedding. |
No. | 21 |
---|---|
Poster Title | Computation harvesting from nature dynamics for predicting wind speed and direction |
Authors | Takumi Aita, Hiroyasu Ando, Yuichi Katori |
Abstract | Complex dynamics arise in natural phenomena due to nonlinear interactions among their components, offering a potential computational resource. In our sensor-rich society, it has become feasible to capture and leverage these natural dynamics. For example, surveillance cameras indirectly record plant movement, serving as sensor outputs that reflect the influence of the wind on the plant. In this context, based on the principles of physical reservoir computing, we introduce a method for estimating wind speed and direction using data from naturally occurring sensors in movies. We validate its efficacy through experimentation. |
No. | 22 |
---|---|
Poster Title | Temporal information processing using Mahalanobis distance of reservoir states |
Authors | Hiroto Tamura, Kantaro Fujiwara, Kazuyuki Aihara, and Gouhei Tanaka |
Abstract | Reservoir computing is increasingly recognized as an efficient machine learning method for time-series processing, notable for its low learning cost and reduced training data requirements. Departing from the conventional linear regression readout used in standard reservoir computing, this study introduces a "distance-based readout" method. This method is especially effective for tasks requiring differentiation, such as anomaly detection and classification. First, we introduce the Mahalanobis Distance of Reservoir States (MD-RS) for online time-series anomaly detection. In the MD-RS method, the reservoir's responses to normal time series are fitted to a multivariate Gaussian distribution. During testing, the Mahalanobis distance from this fitted distribution is used as a measure of anomaly. Notably, MD-RS outperforms both existing reservoir-based and the most advanced techniques without reservoirs in this domain. Further, we extend MD-RS for time series classification. In this method, the reservoir's responses to each class are fitted to a multivariate Gaussian distribution, and classification is based on the distance (measured as Kullback–Leibler divergence) between the distribution of each class and that of the response during testing. Our proposed method demonstrates superior performance compared to readouts using linear regression or support vector machines. |
No. | 23 |
---|---|
Poster Title | Revisiting memory capacity of a reservoir computer |
Author | Swarnendu Mandal |
Abstract | Reservoir computing (RC) has been proven to be overwhelmingly successful in handling a wide range of complex tasks in nonlinear science. The root of its exceptional capability to process temporal information lies in the dynamical nature of its reservoir layer. The nonlinearity and memory capacity the dynamical system help to capture the temporal input information. However, being a intrinsic character of the reservoir, it not always easy to enhance the memory capacity. It has been long-standing problem in the traditional RC architecture. Here, I discuss an alternate architecture for RC where nonlinearity and memory capability is separated out from a single layer. Information of the previous reservoir states are manually inserted in in the current states to enhance memory capacity. The result leads to better performance in tasks requiring higher memory. |
No. | 24 |
---|---|
Poster Title | Evolving multi-reservoir network for understanding emotions |
Authors | Anubhav and Kantaro Fujiwara |
Abstract | This work explores a metaheuristic search approach for developing an efficient multi-reservoir network mapping physiological signals, namely electroencephalogram (EEG) signals to emotions. Deep Neural Networks are trending for classification tasks such as emotion recognition. Although they obtain high prediction accuracy, DNNs require an extensive dataset and enormous computational resources for training. Therefore, we examine a computationally inexpensive reservoir computing network as an alternative to DNNs. We develop a multi-reservoir computing network using insights from works such as Yang and Mazon, Anubhav and Fujiwara, where a multi-reservoir network is noted to imitate brain information processing closely. A Genetic Algorithm based search approach is used to simulate different configurations of multi-reservoir networks and evolve an efficient configuration for the emotion recognition task. In each network configuration, a single reservoir represents a brain area, and connections between these reservoirs indicate communication between different brain areas. In implementation, a chromosome in the search algorithm contains information about the number of reservoirs, EEG channels input to a reservoir and the connections between these reservoirs. Using suitable mutation and crossover operators, we aim to understand interactions of different brain areas and their contributions towards emotions. For experiments, we use a benchmark publicly available DEAP dataset consisting of participants' EEG signals while watching music videos. In future, this work can be extended to understand interactions in brain areas leading to a particular emotion or to develop an efficient multi-reservoir network for different applications. |
No. | 25 |
---|---|
Poster Title | Data-driven modeling of time-varying GRN for early disease prediction |
Authors | Yosuke Inoue, Masaki Inoue |
Abstract | In this study, we focus on data-driven modeling of the state equation for a time-varying Gene Regulatory Network (GRN). Assuming that the transition from a healthy to a diseased state is modeled by the destabilization of equilibrium states and bifurcation as a dynamical system, we aim to predict the timing of bifurcation only from data of the healthy state. Additionally, we introduce a demonstration device to visually comprehend the three states: healthy, diseased, and the intermediate pre-disease state during the poster presentation. |
No. | 26 |
---|---|
Poster Title | Introduction to the Data Analysis Function Integrated with GakuNin RDM |
Authors | Ikki Fujiwara, Yusuke Komiyama, Kazutsuna Yamaji |
Abstract | The importance of research software is receiving greater attention in terms of research reproducibility with FAIR data. With the aim to enable non-expert users to easily conduct reproducible data-driven research, we have developed our JupyberHub service that is integrated with our research data management system, namely GakuNin RDM. Technically, we have extended GakuNin RDM so as to enable users to build and manage their own Jupyter containers on our Kubernetes cluster or on the JupyterHubs that have built by the users themselves. From within the Jupyter environment the user can read/write his/her files managed by GakuNin RDM via FUSE. This service is provided to the members of universities and institutions participating in GakuNin, the Academic Access Management Federation in Japan. Unlike Google Colab or Code Ocean, we aim to provide a quasi-persistent environment based on the trust provided by GakuNin. Beyond the JupyterHub functionality, we aim to support GakuNin RDM users to easily utilize HPCs (e.g. supercomputers) via workflow execution services or batch job schedulers. |
No. | 27 |
---|---|
Poster Title | Hippocampal Structures Among Japanese Adolescents Before and After the COVID-19 Pandemic |
Authors | Lin Cai, Norihide Maikusa, Yinghan Zhu, Atsushi Nishida, Shuntaro Ando, Naohiro Okada, Kiyoto Kasai, Yuko Nakamura, Shinsuke Koike |
Abstract | The importance of research software is receiving greater attention in terms of research reproducibility with FAIR data. With the aim to enable non-expert users to easily conduct reproducible data-driven research, we have developed our JupyberHub service that is integrated with our research data management system, namely GakuNin RDM. Technically, we have extended GakuNin RDM so as to enable users to build and manage their own Jupyter containers on our Kubernetes cluster or on the JupyterHubs that have built by the users themselves. From within the Jupyter environment the user can read/write his/her files managed by GakuNin RDM via FUSE. This service is provided to the members of universities and institutions participating in GakuNin, the Academic Access Management Federation in Japan. Unlike Google Colab or Code Ocean, we aim to provide a quasi-persistent environment based on the trust provided by GakuNin. Beyond the JupyterHub functionality, we aim to support GakuNin RDM users to easily utilize HPCs (e.g. supercomputers) via workflow execution services or batch job schedulers. |